Episode 272 - ManureDB: Updating the Science of Manure, One Sample at a Time - UMN Extension's The Moos Room
00;00;12;22 - 00;00;34;23
Speaker 1
Welcome, everybody, to the Moossroom room. Yes. You are here incorrectly. Do not adjust your audio. This is Emily, I am back. I've been doing a lot of travel here. But excited to be back on the podcast today. Not only with Bradley, but we once again are joined by a great guest. Really excited about today's guest.
00;00;35;01 - 00;00;40;22
Speaker 1
We are joined by U of M extension's engineer, Erin Courtis. Welcome, Erin.
00;00;40;24 - 00;00;41;28
Speaker 2
Happy to be here.
00;00;42;00 - 00;01;02;29
Speaker 1
Yes, we're excited to have you here, too. So we'll dive in a little more to Erin's work. As an engineer. And what she doesn't extension. And we're going to be talking about a really cool, database she's working on. But first, if you've listened for a while, you know that we have some business. We need to attend to first, as we do have a guest.
00;01;03;01 - 00;01;23;01
Speaker 1
So, Erin, we have some questions. We ask all of our guests. We keep a tally of all the answers. It is purely just for fun. Don't let Brad, pressure you into thinking there are right or wrong answers. So your first question is, what is your favorite breed of dairy cattle?
00;01;23;08 - 00;01;23;22
Speaker 2
Holstein.
00;01;23;27 - 00;01;30;03
Speaker 1
Holstein? Good answer. Brad is disappointed. I am not.
00;01;30;05 - 00;01;34;15
Speaker 3
Exactly. Exactly what? But we'll accept it today.
00;01;34;17 - 00;01;35;17
Speaker 2
Yes.
00;01;35;20 - 00;01;59;15
Speaker 3
Okay. Well, good. Let's, If you haven't listened in a while, we'll go through the tallies. Holstein still at the top with 25 jersey 20. So not too far behind. Brown Swiss 13, Montpelier. Three Dutch Belted three, Guernsey three, Ayrshire three, milking Shorthorn two and Normandy two.
00;01;59;17 - 00;02;00;07
Speaker 1
All right.
00;02;00;13 - 00;02;03;16
Speaker 3
So we have a wide variety of of choices.
00;02;03;16 - 00;02;21;06
Speaker 1
Yes. And now as I'm listening to these tallies, I'm really realizing just how many guests we've had on this podcast, which is pretty cool. But, we'll get to our second question now. So, Erin, you maybe have a hunch what the second one is, and that is, what is your favorite breed of beef cattle?
00;02;21;08 - 00;02;26;27
Speaker 2
Oh, that one's a little harder because, I'm going to go with Herford.
00;02;26;29 - 00;02;27;23
Speaker 1
Herford?
00;02;27;23 - 00;02;29;29
Speaker 3
Yes, that is.
00;02;29;29 - 00;02;31;01
Speaker 1
Probably agrees with our.
00;02;31;05 - 00;03;05;23
Speaker 3
Answer. That is correct. That is correct. We are gaining now. So Black Angus is still in the lead at 18 Herford Close second 14, then Black Baldy five. Scottish Highlander five. Charlie four. Red Angus four, Shorthorn three, Simmental two, Belted Galloway two, Brahma two and all with one stabilizer. Kelvin, Keanna and Alora Jersey Belgian Blue Brangus, Piedmontese white pak and miniature Scottish Highlander.
00;03;05;25 - 00;03;26;08
Speaker 1
Got a wide variety there on the beef side. Of course there are more options I suppose too. All right, so now that we have, you know, the important business out of the way, we we can start to dive into the other stuff here. So, Aaron, a big reason we wanted you to come on today was to talk about this new project you've been working on.
00;03;26;08 - 00;03;48;18
Speaker 1
So, like I mentioned, Aaron is, an extension engineer for us at U of M extension. And she does a lot of work on kind of the livestock side facilities type stuff. Probably way more stuff I'm forgetting, too. But she has been working on this really neat, dashboard database. What have you, called manure?
00;03;48;18 - 00;03;54;10
Speaker 1
DB. Which I would say the name is pretty self-explanatory.
00;03;54;13 - 00;03;58;23
Speaker 2
We think so. Yeah, we we thought long and hard about that. Right? Right.
00;03;58;28 - 00;04;09;08
Speaker 1
Yeah, yeah. So, Yeah. Aaron, you can maybe tell us just a little more kind of the surface level. Short answer. What is manure? DB.
00;04;09;10 - 00;04;33;27
Speaker 2
Sure. Well, while the product itself seems fairly new, it has been a project that's in the works for the last 4 to 5 years. It's the result of a project that was led by Doctor Melissa Wilson, one of my colleagues here at the University of Minnesota. And then we had a wonderful grad student, PhD student, Nancy Baughman, that was also a instrumental, developer of this particular project.
00;04;33;29 - 00;04;59;05
Speaker 2
But manure DB arose from this idea that, we're in extension. We're often asked, you know, what's the what are the characteristics of a given type of manure? You know, is it different between this region? Is it different between these stages of production? So the project was really born out of some of those extension based questions. We as, you know, are researching side gigs.
00;04;59;05 - 00;05;26;18
Speaker 2
We have some manure data, at our disposal. But it seemed like there was this wealth of information out there in the, on the part of analysis labs that look at thousands and thousands and thousands of manure samples every year. And so we we wondered to start with, could we aggregate all of that data together? Adds magnanimity to it.
00;05;26;21 - 00;05;51;04
Speaker 2
But try and retain some of the information behind the samples and then and then make that more broadly available. And so that's how manure DB was, was born. It's came through with some USDA funding and we're continuing to work on this. And our CHS is now providing some additional funding to keep it, to keep our research related to it going.
00;05;51;06 - 00;06;02;13
Speaker 2
Yeah, we've been able to, with the help of many, many labs and a stakeholder advisory committee, grow this into what you see now at Minority View. Amanda.
00;06;02;15 - 00;06;20;28
Speaker 1
Well, that was a great little intro. Perfect commercial for it. Aaron. So, yes, this is something, Aaron. Kind of introduced it to our livestock team, a few months ago at one of our meetings. And so, you know, I've had a little bit of a chance to kind of poke around in it and see what it is.
00;06;20;28 - 00;06;50;11
Speaker 1
And, you know, the thing that really gets me is that, there's just a lot, but a lot in a good way. You divide out data by species type, which, you know, I think is the real key here. I'm sure that was kind of number one important thing. You know, but we can also look at things like years, you know, region of, of the country that we're in, you know, the moisture designation of the manure itself.
00;06;50;13 - 00;07;06;18
Speaker 1
And I just think, you know, things like bedding type, all of that. Right? Because we know all those things are going to influence those numbers, right? Year to year. What the weather was like, all those things matter. So, yeah, just as I look at it, there's there's a lot to see and a lot to check off.
00;07;06;18 - 00;07;18;11
Speaker 1
But I do enjoy all the options to really customize it. So I don't know if you want to speak more to some of those customizations or what some of the ideas were there. Aaron.
00;07;18;14 - 00;07;54;05
Speaker 2
Sure. Well, right when we first started, it was, you know, what is swine manure? What is beef manure? What is dairy manure. But then as we started to receive and samples from labs or actually back up a step as we started to work with labs, right? Every lab has a different labs. The middle farm for the for the manure samples that are coming in, and depending on the region of the country and the clientele that those labs serve, they, they breakout animals or manure types or even organic amendment types into umpteen different categories.
00;07;54;07 - 00;08;31;12
Speaker 2
And then we also, whenever any one of us submits a sample to allow, we may add additional descriptors to a sample. Right. Like this is from the gestation barn or this is from the the calf barn. And so we have additional what we call metadata behind each sample as well. And so when we first started it was a very iterative process where with each lab that we became partners with, we had to go back and say, are we adding more descriptors to this manure or are we adding additional types of data?
00;08;31;14 - 00;08;57;18
Speaker 2
And basically add those into our what we call a data template, and then it just broadens the database even more. Now, every single data data sample or every single manure samples, results that are in there doesn't necessarily have all of those descriptors behind there. So we've had to figure out some ways to say, where are we comfortable grouping data and where are we now.
00;08;57;20 - 00;09;26;03
Speaker 2
So one example is that we have many different categories of of dairy cattle based on generally stage of production. But we do round those all up into a common dairy category. But you do have the option to dive down a little bit deeper if, if so desired. We also then another one that's been a little more, interesting but challenging too, is, you know, how do you characterize manure?
00;09;26;05 - 00;10;06;07
Speaker 2
Right. We, engineers have, definition of, of manure, of manure type based on solids content. And that's generally been our, our cutoff points for if we characterize manure. But every lab as, has maybe, just simply a user defined checkbox as to whether it's solid or liquid. And, so we've also been talking about and figuring out ways to group those types of data together on the on the site where there's only a couple points where we actually insert, right, some, some categorization and solid and liquid, we do, add that definition.
00;10;06;07 - 00;10;20;08
Speaker 2
And if it wasn't provided with the sample and that's based on solid or moisture content, depending on how you look at that measurement. And that's the main one that we, that we add in after the fact.
00;10;20;10 - 00;10;41;17
Speaker 1
Yeah. I just again, the level of detail is really something. And, and I also know this is something we had talked about that sometimes with that detail. Right. You maybe only have 1 or 2 data points that fulfill that. So and you had mentioned at the beginning that you guys also thought a lot about privacy. So, you know, on this show we've talked about Fin Bean before.
00;10;41;17 - 00;11;09;11
Speaker 1
That's the university's Big farm financial database. And I know they have some parameters around, you know, if we only have X number of data points from this county, we just don't include it or we combine it with another county. So it can't be sussed out by people. Do you have any sort of those types of, you know, guardrails in place that if you only have X number of samples, you maybe need to search for multiple states to find that information?
00;11;09;13 - 00;11;35;29
Speaker 2
Yeah, sure. We have a couple of, I would say safeguards in place for anonymity purposes and for the and for our project partners, the labs as well. Right. We first of all, when the labs provide us, let's say, a print out of the data, we're not asking for any personal identifiable data. We're not asking for the client names, let's say associated with each sample.
00;11;36;01 - 00;12;00;24
Speaker 2
And at most, what we're asking for, as far as the location associated with the sample, is the first three digits of a zip code. Even then, not all samples have that level of information. And when that's missing, we associate the sample with the labs location, that region. And that's, as far as we go, as far as fine tuning the location for any given sample.
00;12;00;26 - 00;12;22;24
Speaker 2
But, as you mentioned and as, as those examples, let's say from Fin Bean or even, you know, the, the census data, if, as we're looking at data and as before, we even put it into the database, we do make sure that there is more than five samples for a given for a given category for it to be even uploaded.
00;12;22;27 - 00;12;27;02
Speaker 2
If we don't have that, we don't even upload it into the database.
00;12;27;05 - 00;12;48;01
Speaker 3
So tell us, Aaron, a little bit about what what what are we seeing here in the Midwest at least, or compared to other regions of, of the US as far as some of these key components on what's in the manure? You know, I, I've just been poking around a little bit and looking and it seems phosphorus is probably one of the higher, components in the manure.
00;12;48;03 - 00;12;55;02
Speaker 3
Nitrogen, obviously sulfur. So what what what do we see? What what are we what are we looking at in the video?
00;12;55;08 - 00;13;15;00
Speaker 2
We look at whenever we look at these manure samples and we look at them by region. If we're only looking at them from those broad categories of animal type, we do see differences between different regions of the US for any given animal type. And Nancy Bull Baum and Doctor Nancy Bull warm and did a nice synopsis of this as part of her thesis as well.
00;13;15;02 - 00;13;41;29
Speaker 2
But if we're just looking at it by animal type and we're looking at a given manure component, let's say nitrogen, we can will often see some very large differences between the Midwest region here. And for example, maybe this, the southern regions or the southeast region. And, well, we don't have all the metadata behind those given samples. We don't know the exact, storage type.
00;13;41;29 - 00;14;06;15
Speaker 2
Right? We don't know everything behind them. We can make some generally educated guesses. Right. If in the Midwest region here, a lot of our manure systems, at least for swine and even more, growing on the beef side, right, are, deep pit systems which are going to have a more concentrated manure, with, some of our dairy systems.
00;14;06;15 - 00;14;27;28
Speaker 2
But then as we look to the South with more of a lagoon based manure storage system, those manure nutrients become more and more dilute. It doesn't actually mean that there's less of those nutrients in the manure, but on a concentration, if we look at the concentration, the amount per mass of water, the amount per mass of everything else, then there is there are differences.
00;14;28;05 - 00;14;54;25
Speaker 2
That's where when we look at this information, one of the one of the considerations that that we look at from both, an engineering or an application standpoint as well as a research standpoint, is are we looking at these concentration zones on what we call a wet basis or an as is basis that, you know, that sample that you collect from the from that manure, that beautiful sample and that you send to the lab, that would be that as is basis.
00;14;54;27 - 00;15;15;11
Speaker 2
And so it's that's reflective of what we're applying. That's a reflective of what we're then applying. And really that's what this database is built for that as applied. But if we want on a research from a research perspective, for example, to maybe do some more examination or comparison of manure, that's when we might look at those manures on a dry basis.
00;15;15;11 - 00;15;28;06
Speaker 2
How how many nutrients are there for the given amount of solids in that. And the the database does allow us to look at, some of those, look at those nutrients in those different from those different perspectives as well.
00;15;28;08 - 00;15;51;08
Speaker 3
If I'm a farmer and whether it's you have swine manure, poultry manure, beef and dairy, how might a farmer look at this data or if they log on to the website and see how can they use this information, or what what can they glean from it to help in their operations?
00;15;51;11 - 00;16;17;18
Speaker 2
Well, I think the first thing to recognize, if you log on to the site and start digging around and looking at dairy or beef manure, for example, is I hope that you recognize that there's a huge range of concentration values for these manure, which we never wanted this database to replace regular manure or something. So hopefully those that variation in that data continues to encourage regular manure sampling.
00;16;17;23 - 00;16;43;21
Speaker 2
But there are those situations when we simply don't have a manure sample. If we're looking at building a new operation, we don't have animals yet. In an operation, we still need manure, nutrient, data in order to put those nutrient management plans together, to put those proposals together for, local or state, sometimes even federal entities, right, to get that permission.
00;16;43;23 - 00;17;10;05
Speaker 2
And so traditionally, we've gone back to a couple of, let's say, key books, key standards as far as what these manure nutrient contents are. But, those are 20 some years old. And so that was another driver behind this project is can we dynamically update those standard values in a way and reflect what is changing in the industry and thus changing these manure nutrients?
00;17;10;05 - 00;17;39;11
Speaker 2
And so that second new use of this information is if we need some standard value that's reflective of the area, that's reflective of that animal type, this gives us that concentration or a nutrient content to work with for that, for that planning purpose. And then I think the third one, which is maybe a little more distant as far as regular use, but that is looking at looking at over time what has been changing or what are we seeing in terms of changing.
00;17;39;11 - 00;18;11;25
Speaker 2
Now, a farmer has that firsthand knowledge of what's behind the scene has been changing. This database would be more of that broader perspective, right, of of what's been changing in a given area. So and that's not just for the benefit of farmers. That's, that's in a way gives everyone this common playing field to look at manure, not, researchers, those that are putting together all of these models, assessing, you know, state level, nutrient movement or region level nutrient movement.
00;18;11;27 - 00;18;23;29
Speaker 2
We really wanted something that was like kind of like a fair shake across the board for all of these different uses. And so that we're all using similar information versus picking and choosing, for different purposes.
00;18;24;05 - 00;18;56;11
Speaker 3
You know, I just go through it and, well, not all manure is manure. It is very different across species. It's kind of interesting to look at some of the other either swine or poultry, even horse manure in there. It's very different in the components, whether it's phosphorus, potassium or sulfur in there. It's it's quite fascinating to see the differences that most people think that, oh, I'm going to land, apply poultry manure and get the same effect as dairy or beef manure.
00;18;56;11 - 00;18;58;07
Speaker 3
And that's probably not the case at all.
00;18;58;09 - 00;19;24;13
Speaker 2
No. Definitely not. And, you know, we there's manure. But as you start looking at some of these other animals, we, we recognize those of us that work with manure regularly that, that those samples for animals that have a lot of bedding. Right. It's not going to be just a pyramid or sample. It's going to be that bedded manure in which, drastically going to change those concentration values and, organic components and stuff as well of those manure.
00;19;24;15 - 00;19;48;08
Speaker 2
And so, there is going to be some still some interpretation behind the samples and why the samples are as different as they are. But, hopefully it sparks more of these types of conversations too, as to why why manures are different and why not all manures are equal. I guess what's also been fun is, again, we went into it with those main animal species in mind.
00;19;48;08 - 00;20;21;19
Speaker 2
Right? But as labs provided us, the different and different samples that they had analyzed as manures, it's amazing how many examples for of worm manure we have. Right. I think we even have some elephant samples. I'm not sure we had enough to make it into the database, but we have some different animals, in our database as well, which is, from an extension perspective, actually very useful because those we often actually get calls for, you know, what is elephant manure or what is bison manure.
00;20;21;19 - 00;20;53;08
Speaker 2
Concentrate for example. Right. That's not something that's generally published. But now we and anyone else really, hey, that's not on the public dog database. We would, recommend that you just give us a call, because we might be able to find some of these lesser known manures for you. A challenge that we've been grappling with is, we there are a lot of other organic amendments as well that are in way treated like manure, but they're not maybe necessarily from a, from an animal to start with.
00;20;53;08 - 00;21;16;05
Speaker 2
And so at what point do we where do we draw that boundary as far as other organic amendments. And so that's been a just an ongoing conversation as to how we, you know, how we handle that, but we're trying to stick to animal based, products or byproducts and, stick to the US just because of how it is developed.
00;21;16;05 - 00;21;47;18
Speaker 2
But it's it's been fun and it's been interesting to look at that. A good, let's say case study or an example is recently I was asked, what's the difference between, regular dairy manure and digestate from an anaerobic digester. And so I was able to use the database. And for a given animal type in a given region of the US, look at concentrations of dairy manure and then look at concentrations of digestate.
00;21;47;18 - 00;22;08;20
Speaker 2
And for the dairy, I looked at anything that wasn't solid, essentially. And then for the digestate, we do have a category in there now, very different number of samples. And that needs to be considered. When we look at the at the data output from there, it was comparing several thousand, close to 10,000 samples of dairy manure to 48 samples of digestate.
00;22;08;22 - 00;22;37;11
Speaker 2
But, you know, just from that, just that peek into it, we could see that, you know, some of these concentrations that we maybe are worried about, really aren't that different. And that kind of proves some of or justifies that explanation that a digester really is about changing that organic matter. It's not about changing the nutrients. So gives us it gives us some data behind some of these conversations that we're having, especially as we look at alternative manure treatments.
00;22;37;14 - 00;23;05;05
Speaker 1
Well, and I like that it allows you to do a lot of different things. Right. Like you were just talking about we can do some comparison pieces. You know, for me, my first thought was like, this could be used for benchmarking or, you know, as people are thinking about nutrient management plans or whatever. You know, I just appreciate that this is a tool that can be used in a few different ways, and also by a lot of different people, like producers, researchers.
00;23;05;05 - 00;23;28;01
Speaker 1
You know, you kind of mentioned that, and I just feel like sometimes with these types of databases or tools, they're really for one type of person. But this is really a tool that I see a lot of people using, because the way it's organized, the way you can customize the search. And yeah, just because as we were getting in here today, my first thought like, well, you know, what's what's the why?
00;23;28;02 - 00;23;40;03
Speaker 1
Like, why do we have this, what's the use of it? And, and I see multiple uses, right. And used in a lot of different ways for a lot of different people. And I imagine that was part of your goal in designing the whole thing.
00;23;40;06 - 00;24;14;10
Speaker 2
It was. And it's really, really a, an outcome of having a great stakeholder advisory committee that walked with us through this project, providing providing feedback throughout the way, not only feedback input, first of all, and then feedback. That advisory committee included, local vocal consultants, crop consultants, right. Or those that do a nutrient management planning. It included the labs, right, to make sure that we're meeting their needs as well, or providing a useful product back to them.
00;24;14;12 - 00;24;40;16
Speaker 2
And the benchmarking is one of those useful projects products back to them. And then it included sort of data scientists, I would say, or researchers who do, some more of that broader modeling. Right. They need data from a whole region. And so this was one of their they provided some input. That's an example. There is if you look at there's reference guides or little disclaimers in a way explaining the data.
00;24;40;16 - 00;25;06;17
Speaker 2
And that's for those for those researchers. Right. So that they have a, piece of documentation to say what it is, what it is. And, whenever they go to share their results based on this data. And then, we also had, you know, NRCs and some more of that, agency involvement as well. Another key partner is the Manure Analysis Proficiency Program, which is based out of, of Minnesota here.
00;25;06;17 - 00;25;15;07
Speaker 2
But they work closely with labs on analysis proficiency. And so, again, a nice partner to work with as we as we round this out.
00;25;15;09 - 00;25;16;11
Speaker 3
So that's manure.
00;25;16;11 - 00;25;19;01
Speaker 2
We've I could talk manure all.
00;25;19;03 - 00;25;21;23
Speaker 3
Exactly. That's right. Exactly.
00;25;21;25 - 00;25;25;28
Speaker 1
We could talk crap all day. Right. Yeah. I wasn't.
00;25;25;28 - 00;25;26;27
Speaker 2
Sure where I could go.
00;25;26;27 - 00;25;33;16
Speaker 1
With. No, no crap is probably as far as the.
00;25;33;18 - 00;26;00;05
Speaker 3
Well, I think it's very interesting that we can find that we producers can use it. Consultants can use it to really look at what's happening and what they might be able to use this data for in in daily decisions. Tell us a little bit about what are some of the other projects you're working on. You know, Erin, you're very into sustainability and working with farmers.
00;26;00;05 - 00;26;03;14
Speaker 3
So what are what are some other things you're working on?
00;26;03;16 - 00;26;26;04
Speaker 2
Yeah, a lot of my other projects are, like you say, focused on sustainability. And the lens that I try to take to those those sustainability projects is how do we start at the farm level in both talking about sustaining bility and sustainability metrics, or putting some some farm context to that, and then moving into some more of that measurement realm.
00;26;26;04 - 00;26;51;14
Speaker 2
And the focus of my research projects on this sustainability topic is, how can we add some more techniques to get farm specific measurements that relate to sustainability metrics like greenhouse gas emissions? And so I think this manure database dovetails really nicely into that overall mission. Right. Because we are talking about farm level measurements that those are the samples behind the database.
00;26;51;14 - 00;27;13;05
Speaker 2
And then and then moving them up. Looking at how can we take a manure sample and maybe get a greenhouse gas estimate from just that individual sample, not say, I'm perfectly there yet, but we that's that's the impetus right behind this type of work is how can we add some more farm specific information to help in our decision making?
00;27;13;07 - 00;27;39;25
Speaker 2
How can we look at, manure sample? No. If and when we make changes that that's that is changing our manure emissions right now with some very simplistic modeling. We don't always capture all of those site specific characteristics that, that a farmer, that, you know, that a farmer, impacts on a, on a daily or annual basis, however you want to look at it.
00;27;39;28 - 00;28;07;16
Speaker 2
And so by providing some more of these tools, can we start to, to add it into some more work conversation. Maybe it's maybe it's a justification, maybe it's a demonstration of, you know, where a farm is at as far as any of these metrics and not saying any, any and all farms need to change. But I think we all need to be part of the conversation that's going on within the dairy supply chain, within the egg supply chain, within the beef supply chain.
00;28;07;19 - 00;28;31;04
Speaker 2
And having some of these tools behind us at the farm level help us be part of those conversations in a more direct way. Well, if I could make one request and that is when you do submit your manure sample. Not all labs participate in this database, but a good number of them do. And it does take a few extra minutes on the part of those submitting the lab to check a few more of the boxes to describe that manure.
00;28;31;04 - 00;29;00;21
Speaker 2
But, I think it's helpful. Other labs do this type of analysis aggregation as well, and it takes a bit of time. I'm guilty of not fully filling out those forms all the time, but, you know, in a way it's a way to support some more transparency in, in the ag industry, in the manure industry. But but do so in a protected way where the, the data becomes an anonymized and aggregated and, and still supports then development and better use of this manure data.
00;29;00;24 - 00;29;01;24
Speaker 2
So please fill out your form.
00;29;01;25 - 00;29;10;15
Speaker 3
Exactly. Get get your forms, fill them out. So there's lots of good information. So everybody can use that for future projects and data matters.
00;29;10;17 - 00;29;11;14
Speaker 1
You name it.
00;29;11;17 - 00;29;14;02
Speaker 2
Data matters. Yes please. Pretty please.
00;29;14;05 - 00;29;16;26
Speaker 1
Please. We need your data.
00;29;16;28 - 00;29;34;24
Speaker 2
Well, and we hope that we recognize that data is valuable. And and we hope that this this product, this database is has some value going back to, to all the different users and that, that that extra time to fill it out makes a difference to you too.
00;29;34;26 - 00;29;52;17
Speaker 1
All right. Well, I think we'll wrap it there. You know, Erin, thank you so much for coming on today and and being a guest and talking manure and and sustainability and, and I'm just really excited about this tool. So thanks again for for coming on and chatting with us about it.
00;29;52;25 - 00;29;54;15
Speaker 2
My pleasure. It's been a great time.
00;29;54;18 - 00;30;23;22
Speaker 1
So if you're interested in learning more again you can find the manure db database at manure db dot any edu. So that's DB as in database manure db. Any edu. We'd love for our listeners to check it out and let us know what you think. And if you have, you know, questions, concerns or scathing rebuttals, you can email those to the Mus room at Umkc.
00;30;23;22 - 00;30;44;12
Speaker 1
Edu. You can also call and leave us a voicemail at (612) 624-3610. And we look forward, to having you back on another episode. Erin, so we can talk more about some of this any time. All right. Thanks you. Thank you all for listening. And we'll catch you next week.
00;30;44;15 - 00;30;49;28
Unknown
Bye bye. You.
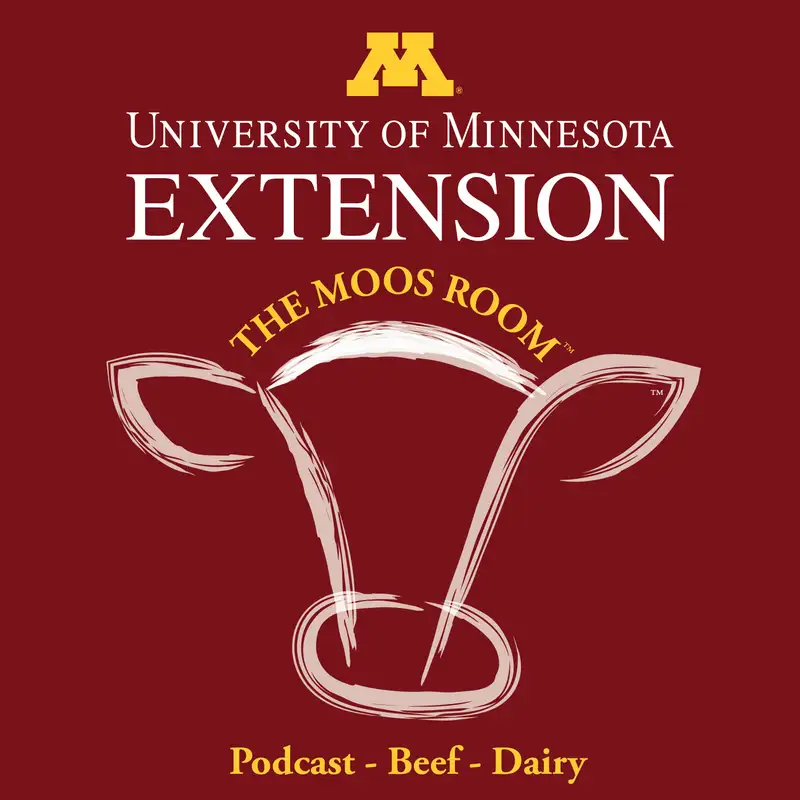